Soil analysis is a vital component of nutrient management and its purpose is to assess the status of available nutrients for crop growth and monitor any changes in nutrient status due to agricultural practices. This is necessary to ensure optimum agricultural productivity and to reduce the incidence of nutrient transfer to waterways and other ecological zones. Regular soil analysis on a period of every 3-5 years is recommended (PDA, 2011). Agricultural practises are a major contributor to poor water quality through sediment and nutrient enrichment, understanding the status of soil nutrients is vital to ensure fertilisation regimes do not potentially increase this risk through the over-application of nutrients above the agronomic optimum.
Traditional soil sampling methods involve taking samples along a ‘W’ pattern across the field with around 30 cores taken using an auger to a uniform depth of between 7.5-10 cm (Collins et al., 2012). These cores are composited to a single representative sample and a sub-sample taken from this for analysis (Peter and Laboski, 2013). Whilst W sampling can indicate the overall nutrient status of the field, it can miss vital information regarding the presence of nutrient hotspots or deficient zones due to the generation of a single average value per field and a lack of information on the in-field variability existing between nutrient values. This can mean that inappropriate fertilisation recommendations can be made based on W sampling which may increase nutrient hotspots or lead to a reduction in the nutrients available for crops.
Precision agriculture can be performed by using gridded soil sampling to design site-specific recommendations for nutrient management in terms of variable rates of fertiliser applications. However, it is often impractical to collect and analyse the sampling grids necessary to resolve the spatial variability existing within soil nutrients for farmers and landowners due to the labour and costs involved (Han et al., 2003). Kriging is used in precision agriculture (based on the regionalised variable theory) to interpolate grid data and provide more robust estimates than traditional interpolation techniques as it uses a variogram to assist in the description of the spatial correlation as most soil properties are strongly positively correlated at short distances (Han et al., 2003).
To quantify the degree of in-field variability of nutrient content, 35 metre gridded sampling regimes were used from which to collect samples at the four study sites used for the Blackwater catchment during the closed spreading period. This grid was generated in ArcMap using digitised polygons representing field boundaries from orthoimagery and the function of create fishnets. An example of one such sample grid is shown below in Figure 1.
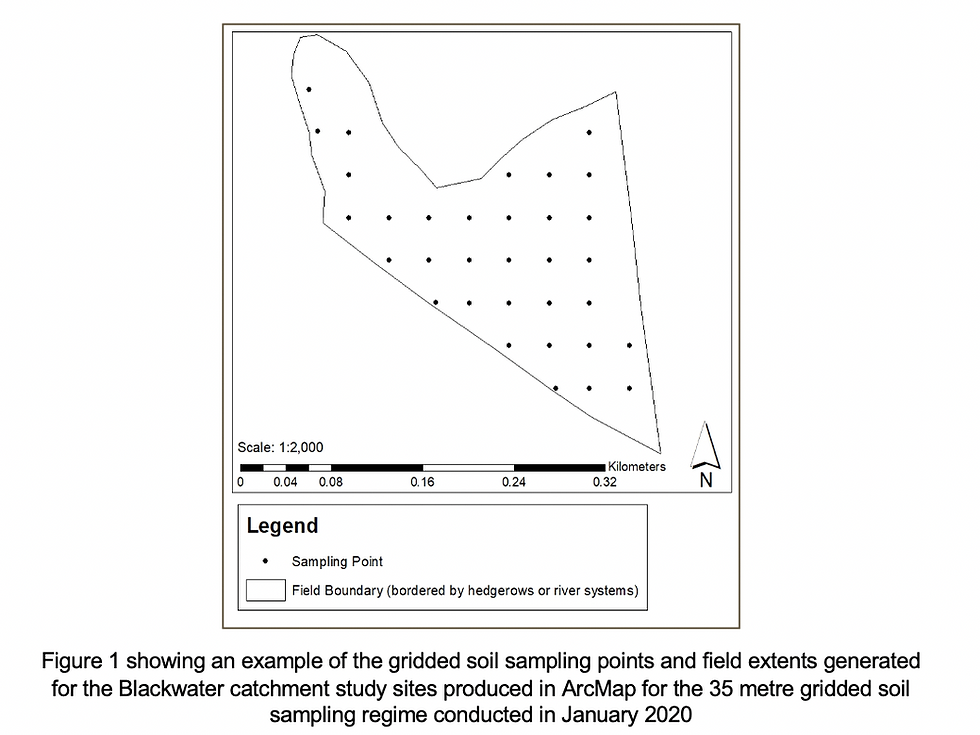
A 35 metre grid interval was chosen as Shi et al., (2002) concluded that this was the best compromise between ensuring efficiency when sampling, whilst allowing good interpolation accuracy for predicting results at unsampled locations. Measurements upon the degree of variability in terms of spatial dependence between soil properties can be conducted using geostatistics, which investigates the extent to which sampled locations will be representative of the unsampled locations and that differences in soil properties occur due to spatial separation (McCormick, 2005). This sampling aimed to establish a baseline value for soil nutrient status, analyse the in-field spatial variability of nutrient statuses, and identify the presence of nutrient hotspots. At each grid point (navigated to in-field using a Leica Viva GNSS GS15 receiver GPS), within a 2 m radius, 20-30 cores were collected at a depth of 7.5 cm using an auger and then bulked to produce one composite sample per point (Theocharopoulos et al., 2001). These soil samples then underwent analysis for pH, phosphorus, potassium, magnesium, and sulphur.
Whilst analysis and interpolation of all sampled nutrients demonstrated that wide in-field variability exists across field sites for soil nutrient content and that W sampling will routinely under predict soil nutrient status, the following presented results focus on phosphorus. This is due to the important role that this nutrient has in influencing eutrophic states in waterways. These phosphorus results are presented as the Olsen P Index, which is the standard agronomic soil phosphorus test in Northern Ireland (Cassidy et al., 2019). The Olsen P methodology (also known as bicarbonate extractable P) is a measure of the amount of bioavailable inorganic orthophosphate in soils (Olsen and Sommers, 1982).
Soil nutrients are classified into an index that is based on plant availability under the UK nutrient management guidelines of the RB209 Nutrient Management Guide. This index system is used by farmers to base their management decisions on for fertiliser applications. The associated index values were calculated based upon the divisions stipulated in the RB209 Nutrient Management Guide, specifically given within Table 3.1 (pg. 9 Nutrient Management Guide (RB209) Section 3 Grass and Forage Crops, AHDB, 2019). For the Olsen P Index, index values of 0 and 1 represent deficient soils, Index 2 (-2 and +2) are optimum values for plant growth, values above Index 3 (ranges from Index 3-7) represent excessive levels of phosphorus in the field. Research has shown that soils that have an Olsen P Index value greater than Index 2, represent a greater risk for detrimental water quality statuses (Taylor et al., 2006).
These results were analysed in ArcMap and using ordinary kriging to produce interpolated mapping displaying the predicted soil nutrient status across the field site. During ordinary kriging, values are kriged at nodes of a fine grid. When kriging on a grid, the variances are small but will increase rapidly towards the boundaries of the support i.e. the field boundaries, where there is no data available beyond this area (Webster and Oliver, 2007). Ordinary kriging variance is the sum of simple kriging variance plus the variance from an estimate of the mean (Webster and Oliver, 2007). However, it must be noted with kriging and interpolation methods that the reliability of this depends upon how accurate the phenomena’s variation is represented by the spatial model. Webster and Oliver (2007) have shown that sampling and associated mapping is most efficient when it is performed on a regular grid to minimise the maximum kriging error.
This allows each field to be split into management zones based upon their nutrient index values. For Index values of 0 and 1, this indicates the field is deficient in P, index 0 is denoted by a pale yellow and index 1 by a darker yellow. Index 2 is the optimum value and is denoted by a green colour. For indices 3 and higher, this indicates that the field contains excessive P, these values were coloured as an increasing gradient of red, with darker shades indicating higher index values. Figure 2 shows the kriging results produced for site four and the wide range in P values of this field site is evident. Whilst further analysis in terms of local site conditions such as topography and soil saturation conditions may reveal further insight into the reasons for this variation in nutrients, this analysis highlights the large degree of in-field variability that can exist in nutrient indices.
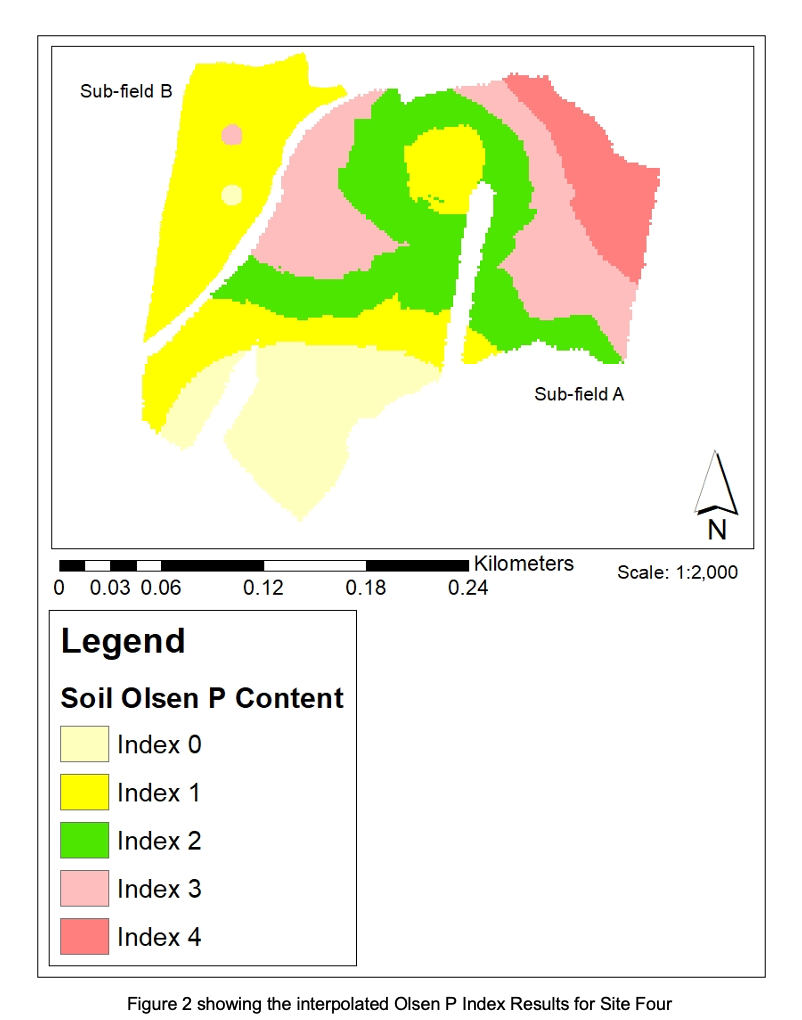
If the above soil sampling results had not been sampled individually and rather a W sampling method was employed, this site would have been classed as Index 2 through averaging and thus ignores both the extensive deficient areas and the widespread hotspots of Index 3 and Index 4 and fertilisers would be inappropriately applied to this site. This can lead to poor agricultural productivity and negative environmental benefits through the continued accumulation of phosphorus within hotspot zones.
This highlights how gridded sampled is necessary to fully appreciate the in-field spatial variability that can exist in nutrient values. It is vital to understand these patterns to apply fertilisers correctly to ensure that areas remain at the appropriate agronomic optimum as specified by the RB209 recommendations (ADHB, 2019). Average singular values produced through ‘W’ sampling do not allow such recommendations to be made and can exacerbate nutrient hotspots or lead to depleted nutrient zones emerging within field sites of variable nutrient concentrations. This shows how each field must be treated individually and it cannot be assumed that all agricultural fields will behave the same due to differences in fertilisation rates, underlying soil and geological properties, differences in management, and variations in topography (Kozar et al., 2002). From a management perspective, this shows why precision agriculture is vital to understand fully the spatial variability existing at a sub-field scale and highlights the challenges to manage grasslands and nutrient balances accordingly.
It also highlights the need however for new cheaper technologies to measure nutrients site-specifically and in real-time such as lab-on-a-chip devices by Smolka et al., (2017). These are still however in their infancy and as such any sampling schemes which move away from a singular averaged value represent a movement forwards in agricultural management, particularly when managing potential sources of nutrient enrichment to waterways.
References
AHDB. (2019). Nutrient Management Guide (RB209). Agriculture and Horticulture Development Board 2019.
Cassidy, R., Thomas, I. A., Higgins, A., Bailey, J. S. and Jordan, P. (2019). A carrying capacity framework for soil phosphorus and hydrological sensitivity from farm to catchment scales. Science of the Total Environment, 687, 277-286.
Collins, H. P., Maysoon, M. M., Brown, T. T., Smith, J. L., Huggins, D. and Sainju, U. M. (2012). Chapter 5-Agricultural Management and Soil Carbon Dynamics: Western U.S. Croplands in Managing Agricultural Greenhouse Gases, pp. 59-77.
Han, S., Schneider, S. M. and Evans, R. G. (2003). Evaluating Co-Kriging for Improving Soil Nutrient Sampling Efficiency, Transactions of the ASAE, 46, 3, 845-849.
Kozar, B., Lawrence, R. and Long, D. S. (2002). Soil Phosphorus and Potassium Mapping Using a Spatial Correlation Model incorporating Terrain Slope Gradient. Precision Agriculture, 3, 407-417.
McCormick, S. (2005). The nature and causes of spatial variability in forage grass production in cool temperate climes. PhD Thesis. Queen’s University Belfast.
Olsen, S. R. and Sommers, L. E. (1982). Phosphorus. pp. 403-430. In: A. L. Page, et al. (eds.) Methods of soil analysis: Part 2. Chemical and microbiological properties. Agron. Mongr. 9. 2nd ed. ASA and SSSA, Madison, WI.
PDA. (2011). Soil Analysis: key to nutrient management planning. Potash Development Association. Leaflet 24.
Peters, J. B. and Laboski, C. A. M. (2013). Sampling Soils for Testing. University of Wisconsin System Board of Regents and University of Wisconsin-Extension, Cooperative Extension.
Shi, Z., Wang, K., Bailey, J. S., Jordan, C. and Higgins, A. H. (2002). Temporal changes in the spatial distribution of some soil properties on a temperate grassland site. Soil Use and Management, 18, 4, 353-362.
Smolka, M., Puchberger-Enengl, D., Bipoun, M., Klasa, A., Kiczkajlo, M., Śmiechowski, W., Sowiński, P., Krutzler, C., Keplinger, F. and Vellekoop, M. J. (2017). A mobile lab-on-a-chip device for on-site soil nutrient analysis. Precision Agriculture, 18, 152-168.
Taylor, M., Drewry, J., Curran-Cournane, F., Pearson, L., McDowell, R. and Lynch, B. (2016). Soil Quality Targets for Olsen P for the Protection of Environmental Values. Integrated nutrient and water management for sustainable farming, Occasional Report No. 29, Fertiliser and Lime Research Centre, Massey University, Palmerston North, New Zealand.
Theocharopoulos, S. P., Wagner, G., Sprengart, J., Mohr, M. E., Desaules, A., Muntau, H., Christou, M. and Quevauviller, P. (2001). European soil sampling guidelines for soil pollution studies. Science of the Total Environment, 264, 1-2, 51-62.
Webster, R. and Oliver, M. A. (2007). Geostatistics for Environmental Scientists. Second Edition, John Wiley & Sons, Ltd. England.
Comments